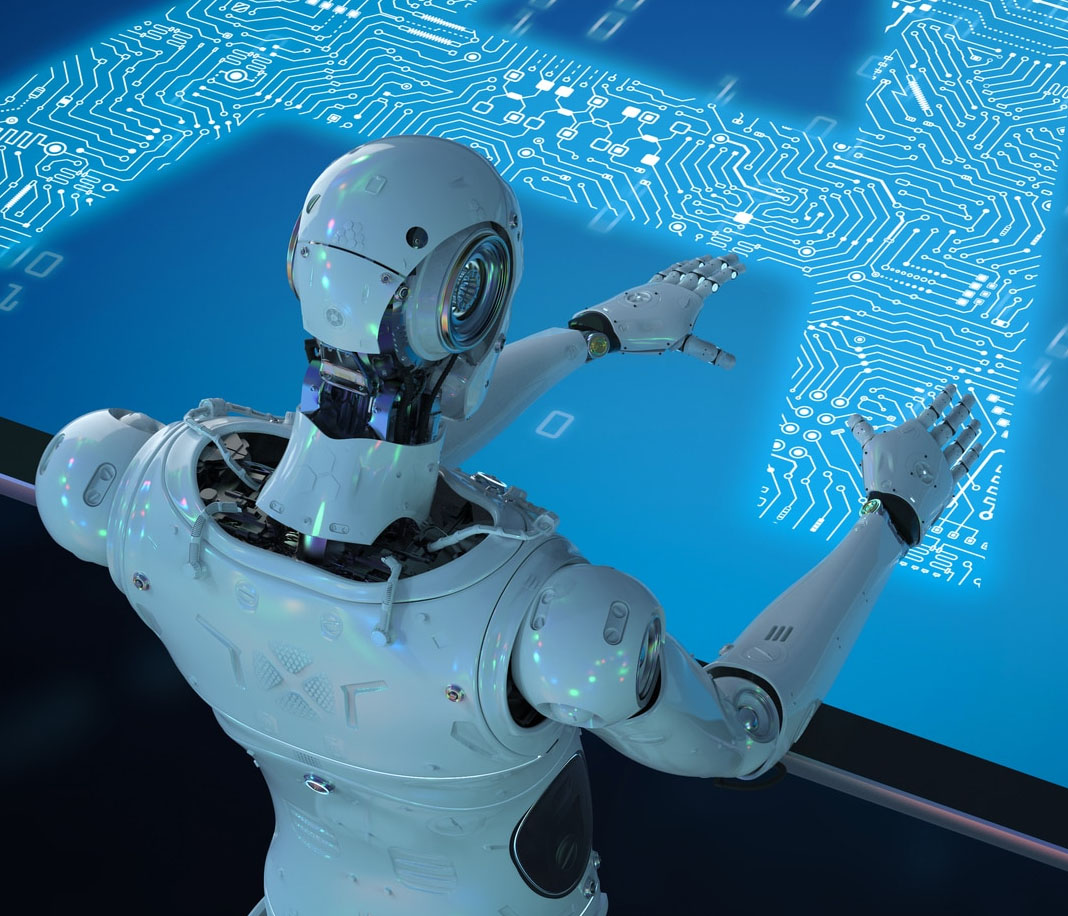
Photo Credit: Getty Images YouTube demonetized two big channels, Screen Culture and KH Studio,...
Photo Credit: Getty Images The nation of Papua New Guinea has implemented a nationwide block on...
Photo Credit: Getty Images Nvidia plans to invest hundreds of billions of dollars in US-made chips and...
Photo Credit: Getty Images Intel Corporation has appointed industry veteran Lip-Bu Tan as its new...
Photo Credit: Getty Images Alibaba's Hong Kong-listed shares jumped 8% on Thursday after the...
Photo Credit: Getty Images Discord, the popular social chat platform, has begun preliminary...
Photo Credit: Getty Images Microsoft has announced that it will discontinue Skype operations in...
Photo Credit: Getty Images Leading AI firms, including OpenAI, Microsoft, and Meta, are turning to...
Photo Credit: Getty Images Amazon has thrown its hat into the quantum computing race, unveiling...
Photo Credit: Getty Images Apple has announced plans to establish a quarter-million-square-foot...
Photo Credit: Getty Images Despite international attention, Chinese tech firms remain steadfast on...
Photo Credit: Getty Images In a strategic move to capture the budget-conscious segment of the...